As an educator and school IT director, I’ve been inundated lately with AI triumphalism. Every morning, my inbox is full of emails from start-ups and legacy tech companies urging me to check out their AI EdTech products. Social media is full of EdTech ‘thought leaders’ talking about AI. The various ListServs I’m on are full of other tech/library people and educators discussing AI.
I’m a skeptical person by nature and I have weathered several ‘this new tech is going to completely revolutionize education’ moments that did not pan out. So, my skepticism about this new development is currently dialed up to 11. One of the things that bugs me the most is that the corporations pushing their AI at me seem invested in making sure people don’t really understand the technology. They use confusing terminology seemingly on purpose, failing to differentiate between different types and purposes of AI. Some seem to be just slapping the AI label on products that only marginally qualify, if at all. In this blog post, I will attempt to clarify my own thinking. That’s one of the major benefits of writing (and one that AI is threatening to nullify for a lot of people). I’ll try to define AI while seeking the nuance that is lacking from the hype machine.
What is AI and how do the hyped up new applications from companies like OpenAI, Google, Anthropic, etc. fit in?
According to Wikipedia, AI is “is a field of research in computer science that develops and studies methods and software that enable machines to perceive their environment and use learning and intelligence to take actions that maximize their chances of achieving defined goals.”
So, it seems like the technology hinges on learning. There’s a term for that…
Machine Learning is the foundation of AI. The goal of machine learning is to design and run computer models that result in computer algorithms for finding patterns in data. This could result in an algorithm that suggests movies you might like, looks for signs of cancer in medical images, predicts stock market shifts, pilots autonomous vehicles, etc. Machine Learning has been around since the 1950s.
ML uses various types of models to analyze different kinds of data. It can be supervised or unsupervised.
- Supervised -> Desired output is already known. Tagged data is used to train the algorithm. For example, a set of x-ray images that all include tumors used to train an algorithm to spot tumors.
- Unsupervised -> Uses unlabeled data. Model learns to identify patterns in the data and group or cluster them. For example: Anomaly detection might be used to find fraud in hospital billing records or instances of hacking in computer network data.
A metaphor that might help understand learning, models, and algorithms: Imagine you are walking in the woods. You are using your brain’s visual processing model to take in and process what you see. You see a group of delicious chantarelle mushrooms under an oak tree. You think ‘Ah, chantarelles grow under oak trees’ (learning). The next time you go mushroom hunting you look under oak trees because your mushroom seeking algorithm has been updated.
That doesn’t really sound like what ChatGPT does though. What’s the difference? With ChatGPT and other ‘large language models’, the learning part has already happened. The LLM is the algorithm that is a result of the learning or ‘training’. In this case, it’s a generative algorithm.
Generative AI is a subset of machine learning. It didn’t get off the ground until about 10 years ago due to limitations of data storage and computational power. GenAI Goes beyond machine learning/data analysis to generate novel content. The input data for training is a huge corpus of text, image, music, or video. GenAI is essentially a prediction machine. It generates the next word or pixel or note based on context provided by previous words/pixels/notes and according to the statistics it has gleaned from its training data.
- LLMs (Large Language Models) use transformer models for training
- Image, sound, and video generation AI uses diffusion models for training
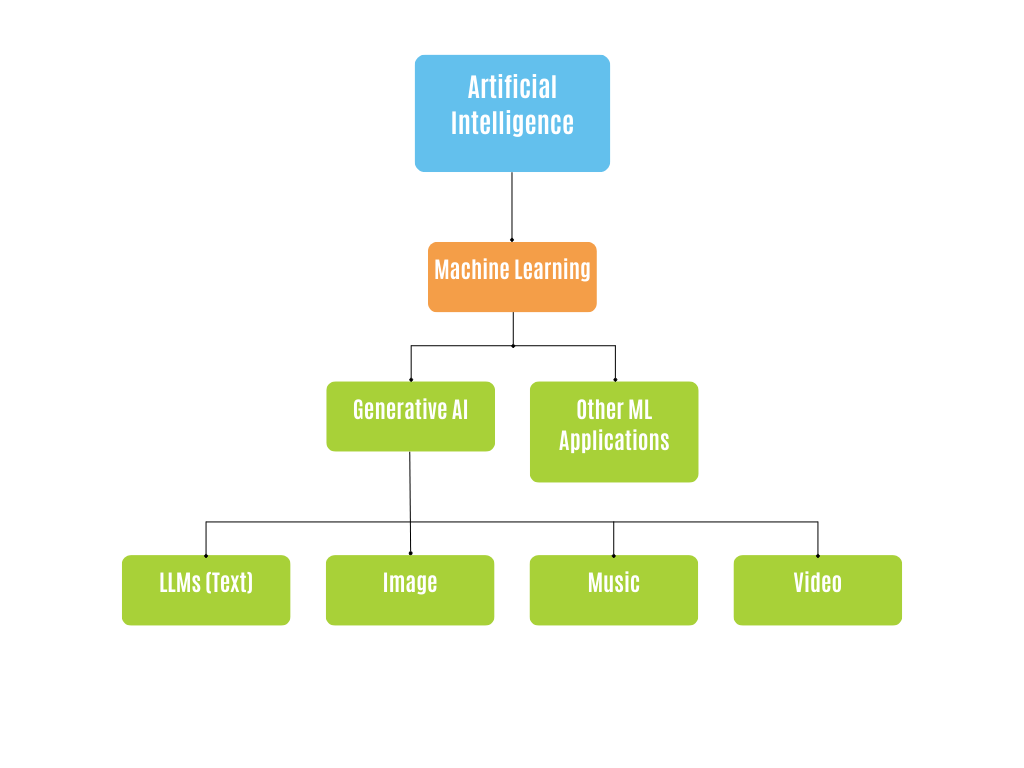
So, Generative AI is an application of Machine Learning. There are many other applications, some that you could argue are positive (enabling scientific discoveries, modeling complex systems, self-driving cars), some possibly negative (use in warfare, surveillance, privacy invasion).
Unlike other Machine Learning applications, Generative AI attempts to mimic the human act of creation. Computer scientists have made GenAI algorithms for creating written text, images, video, and music.
I probably wouldn’t be writing this essay if I thought this was a good thing. So, what’s my problem? It’s not with machine learning/AI in general. As I mentioned above, I think there are good and legitimate applications of machine learning. I dislike GenAI in particular for the following reasons:
- It attempts to create simulacra of human creations for no apparent reason. Why do we want computers to do our writing for us? Or compose our music? Or generate images? Those are all things humans are good at. And, they are things that make humans happy. And, they are things that improve the minds of the humans who do them. Flooding the world with computer generated slop is not a positive. Also, producing the slop consumes massive amounts of electricity and water. Just because we can do something, doesn’t mean we should. I will choose a human-made thing over a computer-made thing 100% of the time. Not because it’s necessarily better in and of itself, but because it is infinitely better if you consider the whole context of its creation.
- GenAI obviates productive struggle. I wrote a whole essay about this so I don’t need to explain here.
- I prefer to do my own thinking and so should other people. Using your brain keeps it sharp. Just like taking the stairs (if you are able) instead of an elevator keeps your legs strong. When people stop thinking for themselves, they will lose the ability to think for themselves. As they progressively lose this ability, they will become proportionally less able to realize they have lost this ability.
- When people can’t think for themselves, it’s easy for unscrupulous people to take power and do bad things. The unscrupulous people probably think this is fine. They might even be the same people who are creating the GenAI. Hmmm…
- Content/material production is not just a one way transaction. It changes the person who does the production in important ways. There is a feedback effect. If I make a pie and share it with you, that is a very essentially different act than buying a pie and sharing it with you. For example: if I let an LLM write my report card comments, I will miss the essential experience of thinking deeply about each of my students. Writing comments is hard but it forces me to get to know my students better, to think about each of them as individuals.
- Something uniquely human and important is lost when we buy pies instead of making them. Or generate poems instead of writing them. Maybe I’m just an old man shaking my fist at an LLM. But, I’m not wrong on this point. GenAI tech is anti-humanist, anti-humane, anti-human.
- They corporations selling AI have to make these things profitable somehow. Expect cost to rise substantially and/or to start seeing ads and creepy data collection introduced into the products. Massive tech corporations like Google and Facebook have had to substantially degrade their products, turning them into creepy data-collecting, advertising machines in order to make a profit. OpenAI and Anthropic won’t be far behind.
One GenAI application I’m on the fence about is AI ‘tutors’. For example, Khan Academy’s Khanmigo. I think these might eventually be useful but Khanmigo uses GPT-4 on the backend. GPT-4 (just like all major LLMs) is trained on stolen data, uses obscene amounts of power and water, hallucinates, and embodies the deep racism, ableism, and sexism of its training data.
No matter how useful AI tutors end up being, the presence of an actual, warm-blooded human being giving a student one on one, focused teaching should not be discounted. If the argument is that this is not possible because public schools are underfunded and teachers have 30 kids in their classrooms, look at fixing that before you turn the teaching over to computers.
In summary, I’m not against machine learning, per se. But I do detest generative AI for a lot of (in my opinion) good reasons. It is, on balance, both unnecessary and actively harmful to the earth, human brains, and the quality of the vast, amazing archive of information we call the internet. Let’s not trade our humanity, our ability to think and create, for a cheap handful of AI slop.